Introduction
Spending on fertilisers and agrochemicals represents a considerable part of farmers’ overall expenditure. By using hyperspectral imaging to detect what nutrients and how much of them a crop needs in the different growth stages, such costs can be reduced. Hyperspectral imaging is the process of creating an image through electromagnetic radiation, carried out by an automated drone system. The use case demonstrates both in potato as well as wheat farms how data from different types of sensors - measuring parameters such as soil moisture and organic matter or climate conditions – combined with spectral data analysis can be used for precise decision-making and optimised crop management. The data gathered enables farmers to predict yields, define specific management zones, and accurately calculate the required fertilisers, herbicides and other agrochemical products. This use case thus delivers an innovative solution to pressing issues in arable farming.
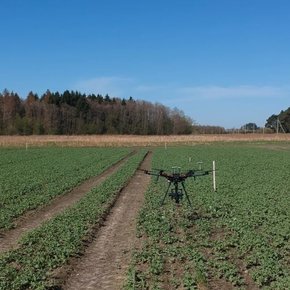
Breakthrough in precision agriculture
Successful agricultural operations depend on field management including crop monitoring for nutrients, irrigation, diseases, and overall plant health. Traditionally this has been carried out by visual examination of crops on the ground. However, these methods are limited and only fighting the symptoms instead of proactively preventing them.
Show more
This use case leverages precision agriculture, artificial intelligence and hyperspectral imaging for optimised crop yield and fertilization while reducing the environmental impact. The key is targeted application rather than whole-field treatment. But let’s not put the cart before the horse and define the underlying concepts before we dive deeper into the matter.
To validate and improve their solution Kristina Šermukšnytė-Alešiūnienė, the Use Case Coordinator, and her team use a test field of 5 ha close to Kaunas, Lithuania to experiment with various cover crops for autumn, different plant protection products, as well as drainage systems are tested. By determining the most resistant mixes of varieties diseases such as winter harness or yellow rust, common nuisances in the Baltics, are avoided. To ensure the comparability of their research results, the team follows a rigid methodology when taking plant tissues to the laboratory for a subsequent analysis. It is not without its struggles, however, as the application of pesticides and fungicides is hampered by adverse weather conditions and outdated agricultural machinery. Hence, farmers need to rely on advisors that know the regional specificities like the back of their hands. Their service includes a web platform for hyperspectral data submission and storage, as well as data aggregation and integration with the GIS-based FMIS AgroSmart for analysis result presentation and delivery. Further integrations with other FMIS (such as 365FarmNet and Akkerweb) are currently explored.
A drone equipped with a hyperspectral camera is able to classify crops through sampling images. Aside from sensors and cameras, the drone was equipped with a spectroradiometer, collecting solar radiance in different wavelengths form the surface. Before take-off, all components need to be calibrated and adjusted to the current light as well as weather conditions. The data samples gathered by the drone, are later integrated into images using a time-stamp method. Abrupt weather changes would affect the accuracy of this data during processing but, fortunately, this was not the case as we were blessed with an Indian summer during our entire visit of this use case. The image filtering techniques are further enhanced by laboratory tests on samples from the exact same location, thus constantly improving calibration and the algorithms used for crop management or yield predictions. This, leading to staggering measurement accuracy yield of up to 80%. Since algorithms can be described as opinions in code, the visualisation of these opinions is the foundation on which advisors and farmers alike can base their decision making. The benefits are usability, rapid assessment, accuracy and consistent results. Unsurprisingly, it is considered a breakthrough in the proliferation and practical application of precision agriculture.
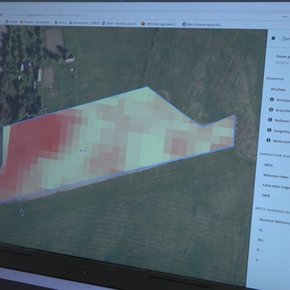
Crop intelligence serving local challenges
Drought is a significant factor in terms of crop yields. Thus, early detection of water related stresses allows farmers to accurately irrigate before the effects result in yield losses. Hyperspectral imaging detects such changes long before it is visible to the human eye. The practical applicability was inter alia demonstrated in a trial with barley, where the development of water or nutrient stress has been detected four days before it was observable with the naked eye.
Show more
Lithuania alone used 167kt of nitrogen, 76kt potassium and 54kt phosphorus in 2018. After a drought hit the Baltic fields early in Summer 2019, this number most likely even grew higher. Even though the situation was dire, this use case could unfold its full potential through their non-invasive remote crop field monitoring and plant assessment which is the cornerstone of their hyperspectral plant analysis services. Hyperspectral imaging is able to detect micro and macro nutritional elements in crop plants from field images and thus enabling them to get a deep knowledge about the respective field. Among the elements analysed by the neural network are Nitrogen, Phosphorus, Potassium, Sulphur, Manganese, Copper, Zinc and Iron. With the help of such insights concerning these major compounds the team can determine if plants. This dynamic mapping of soil improves precision agriculture and decreases environmental impacts since the application of fertiliser is reduced to its minimum.
Another threat can equally be tackled with hyperspectral imaging: fungal pathogens. Fungal diseases are responsible for losses up to 40 % of global agricultural productivity. Moreover, those consequences can have long-term effects on consumers, public health, societies, environments and farmers. Hence, the losses itself inadequately reflect the overall repercussions. The early detection of plant disease in the field allows producers to rapidly treat affected areas before the optimum time for counteractive measures has passed. Apart from mitigating individual yield losses, this method prevents diseases from spreading to neighbouring fields or crops.
52,5 up to 62,6
Yield increase potatoes (t/ha)
7,5 up to 8,6
Yield increase wheat (t/ha)
The possibilities for precision agriculture are numerous and will keep on growing as indexes for each plant species, nutrient or soil property are continuously developed and improved. Currently, many other applications of hyperspectral imaging are being tested in post-harvest quality control, food safety of agricultural products or insect and contaminant detection. Portable devices have become a vital tool for researchers and farmers alike because they deliver accurate data while keeping the costs low. These devices enhance and facilitate day-to-day monitoring processes, and thus create a new paradigm of agricultural efficiency. It is even envisioned to realise database-to-machine connections for automated farm machinery task planning through ISOBUS connections or the configuration of interconnected tools.
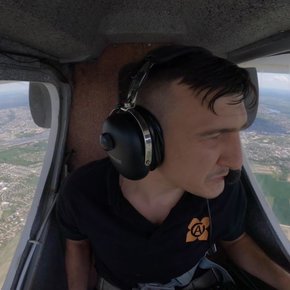
Challenges and lessons learned
The major challenge for this use case is to perform a fast as well as easy plant nutritional state assessment at different plant growth stages and determination on what specific nutritional elements are lacking in which field areas.
Show more
At the beginning of the project phase regular field scanning flights were performed using UAVs such as a heavy lifting drone equipped with hyperspectral cameras, spectro radiometer, GPS tracker and on-board computer. Due to the weight of this equipment the flight time is limited to around 40 minutes. Those automated flights usually take at least 2 hours, depending on the size of the field. That left the team stuck between a rock and a hard place. To respond to that, they developed a functional prototype of a solution for remote wheat and potato nutrient composition assessment based on the same idea but with one crucial advantage: coverage of entire countries within a short timeframe. Instead of the drone, the entire sensing module was mounted on a fixed wing ultra-light (piloted) airplane which was flown over Lithuania while successfully conducting hyperspectral data gathering experiments above targeted winter wheat, potato and other crop fields. In addition to the main research area of hyperspectral imaging, several different spectral sensors by different manufacturers and their data compatibility have been tested, including HSI snapshot cameras and NIR sensors.
229,5 up to 323
Fertiliser cost reduction potatoes (€/ha)
160 up to 224
Fertiliser cost reduction wheat (€/ha)
Another challenge the team had to overcome was limited data computation capabilities. Due to the use of data de-noising algorithms, the dataset size use to expand 8 times during the pre-processing stage, exceeding in-house data computation capacities. An alternative method has been found to reduce noise levels through consolidation of several separate data points into single median-value data units, thus significantly reducing computational requirements while also increasing the accuracy of results at later stages. Additionally, integration with cloud computing providers has been done (specifically Amazon Web Services), enabling utilisation of on-demand computational power when required. Initially used conventional approaches for crop field hyperspectral data processing became challenging when scaling with increasing large dataset sizes. After reassessment redundant processes (raw data de-noising) were discarded and new ones (data point median consolidation) implemented, thus reducing computation power and time requirements, and increasing analysis result accuracy.
After talking to the team, they see great potential in the cooperation and sharing of methods with our arable use cases Precision Crop Management since they analyse very similar things. More obvious is the collaboration with the use case Within-field Management Zoning in terms of common communication standards and data structures for information submission to link sensor information to data infrastructure. Consultations with both use case teams and other external experts helped to identify previously unaccounted plant physiological and environmental factors that are influencing spectral data parameters, such as correlations between specific nutritional elements or spectral data distorting effects caused by shadows cast by plants. Leveraging these valuable insights were and integral part of the development process and provided more nuance in further development planning.
Get in touch with the use case team via the SmartAgriHubs Innovation Portal
Go to portalAchievements, products & services
AI technologies (Machine Learning/Neural Networks) to perform complex analyses of crop field hyperspectral images
Automatic recommendations for agrochemical application through non-invasive, remote sensing technology
Identification of indicators to determine the composition of nutrients in crops
FMIS integration for mapping of micro- and macronutrients in potato and winter wheat plants